Applying Machine Learning to Engineering and Science
Course 2 of 2 in the program Machine Learning, Modeling, and Simulation: Engineering Problem-Solving in the Age of AI
- START DATE April 29, 2024 More Dates
- TIME COMMITMENT 4-6 hours per week
- DURATION 5 weeks
- FORMAT Online
- PRICE $1,469
WHAT YOU WILL LEARN
Learn how the computational tools used in engineering problem-solving are put into practice in course 2 of this 2-course program.
View the weekly schedule here.
This course is also offered in SPANISH (Machine Learning Aplicado a la Ingeniería y Ciencia) in collaboration with Global Alumni.
- Understand why and how machine learning methods may improve engineering problem-solving.
- Learn how researchers make better predictions with missing or sparse data.
- Transfer machine learning approaches developed in one industry to another industry.
- Quantify risk and clarify salient features from data in complex systems.
- Assess conditions when a machine learning approach may not be helpful or worth the extra effort.
WHO SHOULD ENROLL
Industry professionals with at least a bachelor's degree in engineering (e.g., mechanical, civil, aerospace, chemical, materials, nuclear, biological, electrical, etc.) or the physical sciences.
Other technical professionals with a background in college-level mathematics including differential calculus, linear algebra, and statistics.
Programming experience not necessary, but some experience with MATLAB (R) is very useful.
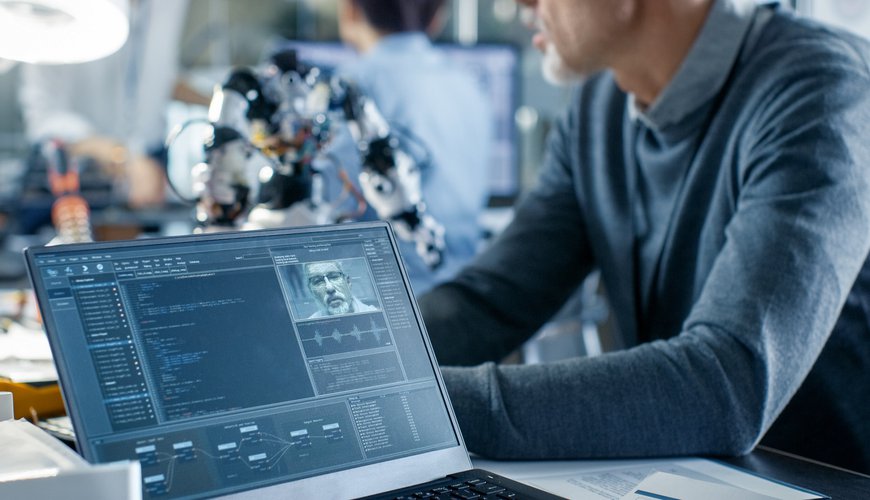
HOW YOU WILL LEARN
-
LEARN BY DOING
Practice processes and methods through simulations, assessments, case studies, and tools.
-
LEARN FROM OTHERS
Connect with an international community of professionals while working on projects based on real-world examples.
-
LEARN ON DEMAND
Access all of the content online and watch videos on the go.
-
REFLECT AND APPLY
Bring your new skills to your organization, through examples from technical work environments and ample prompts for reflection.
-
DEMONSTRATE YOUR SUCCESS
Earn a Professional Certificate and 2.5 Continuing Education Units (CEUs) from MIT.
-
LEARN FROM THE BEST
Gain insights from leading MIT faculty and industry experts.
MIT FACULTY AND INSTRUCTORS
COURSES IN THIS PROGRAM
To earn a Professional Certificate, you must complete both courses in the program. For those who do not want to commit to the full program, courses can be taken on an individual basis. Savings apply when enrolling into the full program.
THE BEST COMPANIES CONNECT WITH THE BEST MINDS AT MIT
Deepen your team’s career knowledge and expand their abilities with MIT xPRO’s online courses for professionals. Develop customized learning for your team with bespoke courses and programs on your schedule. Set a standard of knowledge and skills, leading to effective communication among employees and consistency across the enterprise.
Find out what MIT xPRO can do for your team.
INQUIRE NOW